Is There an AI Digital Divide in Higher Ed?
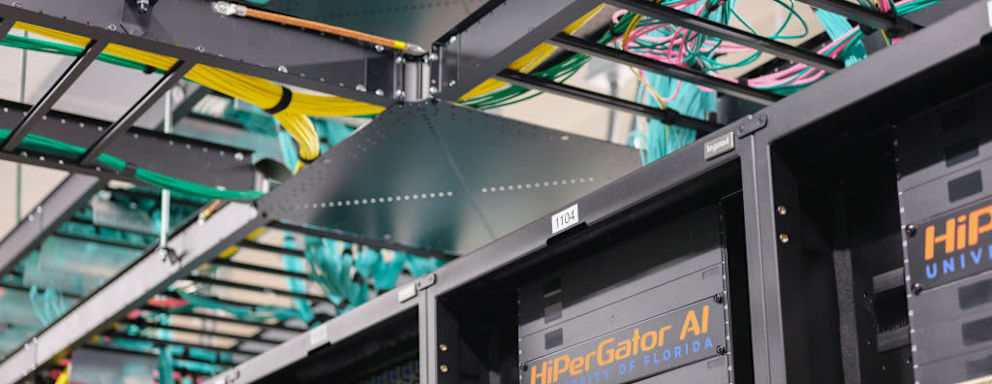
- A new report from Educause reveals how colleges and universities are adjusting to life with artificial intelligence.
- The report examines institutional policies and practices around AI adoption and training.
- Teaching and learning remain a priority, though faculty usage of AI trails student usage.
- Differences in terms of resources dedicated to AI exist between large and small universities.
A new report from Educause shines a light on how — and how much — universities are using artificial intelligence (AI) across campus.
The report, resulting from a survey of college faculty and staff, examines the “digital divide” between institutions of differing sizes, suggesting some are better equipped to accommodate change.
But here’s the truly significant finding: Although 93% of respondents said teaching and learning policies have been impacted by AI or soon will be, only 57% view AI as a “strategic priority.”
When a new technology so pervasively disrupts teaching and learning, the core function of a college, we might assume that technology rises to the level of a strategic priority.
Yet there’s so much more to unpack from this survey, a follow-up to last year’s similar effort from Educause, a nonprofit association whose mission is to advance higher education through information technology.
Overall, the message is that higher education has made great strides in adopting AI, but there’s still plenty of work to do.
Less than a quarter of institutions (22%), in fact, have an institution-wide AI strategy in place. More than half (55%) said AI strategies exist in pockets around the institution. Another 11% said there’s no AI strategy anywhere on campus.
Those who do have a strategy said it pertains mostly to people, not to the technology itself. Key activities include faculty and staff training, increasing access to tools, and ensuring data privacy.
Student concerns remain paramount. The top factor motivating AI-related planning is “the rise of student use in their courses.”
Improving learning experiences and outcomes and preparing students for the future workforce also figure prominently, even though only 22% said AI tools are helping students “learn discipline-specific workforce skills.”
While 84% said they’re worried about plagiarism, respondents noted AI’s ability to help students get answers to problems, edit their work, summarize content such as lecture notes and articles, and brainstorm ideas.
Curiously, when asked how their institution is paying for AI initiatives and upgrades, a plurality (41%) said they didn’t know. Certainly someone on campus must know.
When asked about risks, university leaders said misinformation, the use of data without consent, and data protection were of the greatest concern.
Only 9% said their institution’s cybersecurity and privacy policies adequately address these AI-related risks. And among the 30 respondents who identified as cybersecurity and privacy professionals, zero said their policies are adequate.
Also concerning is the growing division among faculty and staff regarding attitudes about AI, something the report calls a “notably newer result.”
“There is a lack of collegiality between the two sides of faculty,” one individual quoted said. “Our faculty are in two camps — either they love it or they hate it. When they meet, it is often not a positive interaction because of the personal biases toward AI use.”
Another cited “hostility directed at faculty who are experimenting with AI and trying to teach students how to use the tools in creative, ethical ways.”
Still, most respondents remain positive about AI’s potential, noting its ability to automate processes, analyze large datasets, and improve access to educational content.
A central theme of this report is the digital divide caused by AI adoption. One divide pertains to the chasm between faculty and students. More than two-thirds (68%) said students use AI more than faculty even though institutional policies tend to favor faculty training over student training.
Faculty, in other words, have to catch up to their students in terms of AI sophistication.
Another divide, at least potentially, exists between larger institutions (more than 10,000 students) and smaller ones (fewer than 5,000). The survey sought to determine if AI adoption and usage varied by institutional type, perhaps assuming differences exist.
Yet many similarities surfaced.
The survey revealed the two types of institutions “do not differ significantly in their own personal use of AI technologies, their motivations for adopting AI technologies, or their expectations for AI technologies in the future.”
Instead, “respondents from larger and smaller institutions appear to be using AI tools for personal purposes at roughly the same rates.”
For example, when asked about primary motivators for AI planning, 75% of small schools and 74% of large ones mentioned the rise of student use of AI.
Some differences did emerge, however. While just over half (51%) of respondents from large schools cited “faculty enthusiasm” among the reasons for AI planning, only 41% of those from small schools did.
And 25% of those from bigger institutions said AI tools “increase workloads,” though only 18% of their counterparts from smaller schools shared that sentiment. The notion of AI increasing workloads instead of lessening them suggests some misunderstanding or misuse of the tools.
Finally, the biggest difference between the two types of institutions relates to resources. Larger schools seem to be in a better position financially, and perhaps in terms of staffing levels, to accommodate new AI technological developments.
For instance, one-third of larger schools create their own AI applications for internal use, while only 18% of small institutions have that capability.
What does all this mean for colleges? The Educause authors offer some recommendations.
Invest more in student training, they write, matching the resources dedicated to training of faculty and staff. Examine and adopt best practices from other institutions. Do more in-house instead of through outsourcing.
Smaller schools, the report suggests, should connect with peer institutions to share ideas and resources in light of budget constraints.
None of this seems particularly revolutionary, though it’s prudent to be cautious and deliberate. Keeping pace with AI advances is like boarding a moving train.
Sometimes the only option is to make the leap and hope for the best.